Soft Voting Ensemble-Based Approach: for Diagnosing Diabetes Mellitus
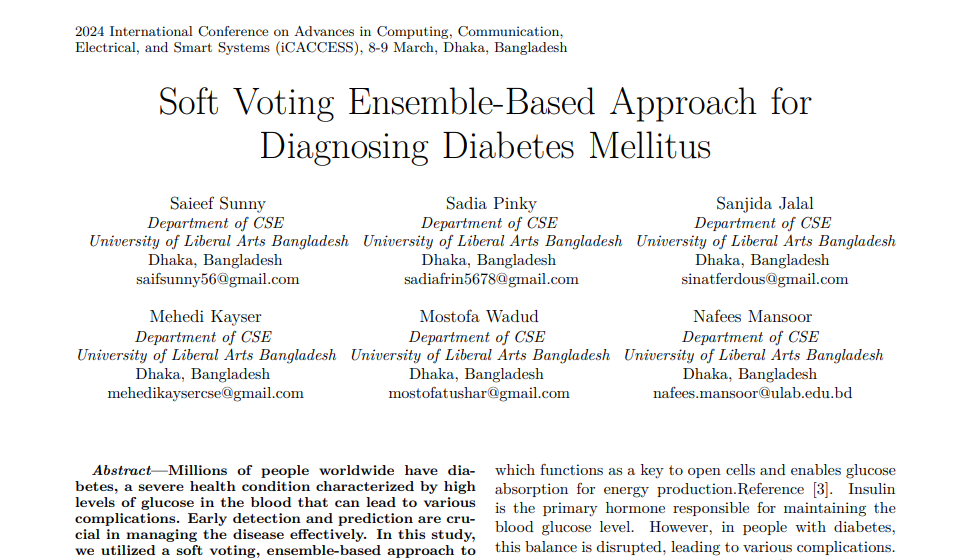
Overview
Abstract—Millions of people worldwide have diabetes, a severe health condition characterized by high levels of glucose in the blood that can lead to various complications. Early detection and prediction are crucial in managing the disease effectively. In this study, we utilized a soft voting, ensemble-based approach to improve the accuracy of predicting diabetes mellitus. The dataset comprised medical records and laboratory analyses of 1000 diabetic patients collected from two hospitals in Iraq. The study utilized an ensemble method with a soft voting classifier comprising Random Forest, XGBoost, and Gradient Boosting to achieve binary classification. The ensemble model achieved an impressive accuracy of 99.50% on the Iraqi diabetes dataset, with precision, recall, and F1-score values of 99.52%, 99.50%, and 99.51%, respectively. This research highlights the effectiveness of ensemble learning in improving the predictive power of machine learning models for diabetes detection.
Key Features
- Severe Health Condition: Diabetes affects millions worldwide, characterized by high blood glucose levels leading to various complications.
- Early Detection and Prediction: Crucial for effective management of diabetes.
- Soft Voting Ensemble-Based Approach: Utilized to improve the accuracy of predicting diabetes mellitus.
- Dataset Composition: Comprised medical records and laboratory analyses of 1000 diabetic patients from two hospitals in Iraq.
- Ensemble Method: Employed a soft voting classifier with Random Forest, XGBoost, and Gradient Boosting for binary classification.
- High Accuracy Rates: Achieved an impressive accuracy of 99.50% on the Iraqi diabetes dataset.
- Performance Metrics: Precision, recall, and F1-score values of 99.52%, 99.50%, and 99.51%, respectively.
Description
Published in the 2024 International Conference on Advances in Computing, Communication, Electrical, and Smart Systems (iCACCESS)
-
22 April 2024
-
IEEE Conference